Updated: Jul 24, 2021
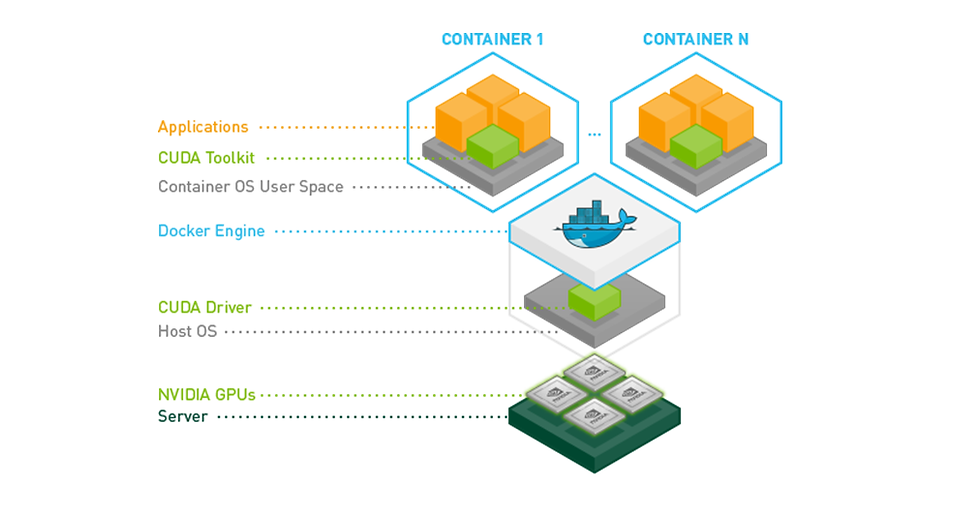
Welcome to my blog!
If we consider different stages to create ML/deep learning models, the first most important step will be to create the environment to train the models.
Today, I just wanted to discuss the different methods available to install and use TensorFlow. Throughout my career, I have delivered most of the deep learning projects for NLP and computer vision where predominantly I have used TensorFlow for deep learning. If you are an aspiring data scientist or ML engineer, you might have come across issues while installing and using TF. My intention here is to provide the big picture of installing and using TF in different possible ways available.
I have experimented with different ways to use TF and have also gone through lots of articles about installing TF. Here, I have created a summary of all that knowledge. I have seen people or articles talking about TensorFlow installation that it's very difficult. The answer is yes and also no. Building TensorFlow from source is definitely not an easy job, but installing TensorFlow for use in your next GPU-accelerated data science project can be done in some simple ways.
Let us start!
Q.1 What is TensorFlow?
TensorFlow is an open-source software library for high-performance numerical computation. Its flexible architecture allows easy deployment of computation across a variety of platforms (CPUs, GPUs, TPUs), and from desktops to clusters of servers to mobile and edge devices.
Originally developed by researchers and engineers from the Google Brain team within Google's AI organization, it comes with strong support for machine learning and deep learning and the flexible numerical computation core is used across many other scientific domains.
Q.2 What are the different ways to use TF?
We can use the precompiled version of the TF provided by google
It can also be build from the source for the specific OS version and other dependencies like Cuda, NVidia driver, CuDNN, and tensorRT.
Q.3 Why build from source?
Following are some of the reasons because of which building TF from source is necessary.
For a normally Python package, a simple pip install -U tensorflow but, unfortunately, TensorFlow is not that much simple in some configurations of OS and dependencies. Sometimes after doing the pip install -U, errors of not founding Cuda or other dependencies will be thrown.
Sometimes we won't get the binaries for latest configuration of the OS version, CUDA version, etc.
Most importantly performance issues. Prebuit binaries might be slower than binaries optimized for the target architecture since certain instructions are not being used (e.g. AVX2, FMA). I have seen a significant amount of performance improvement with the tensorflow which is build from source, with specific dependencies like OS version, cuda version, etc.
Q.4 How to use prebuilt packages with PIP from PyPI?
1.TensorFlow with pip
For latest TensorFlow 2.x packages
tensorflow —Latest stable release with CPU and GPU support (Ubuntu and Windows)
tf-nightly —Preview build (unstable) . Ubuntu and Windows include GPU support .
Older versions of TensorFlow 1.x
For TensorFlow 1.x, CPU and GPU packages are separate:
tensorflow==1.15 —Release for CPU-only
tensorflow-gpu==1.15 —Release with GPU support (Ubuntu and Windows)
For installation of tensorflow with pip just execute following commands
Install the Python development environment on your system
Create a virtual environment (recommended)
Install the TensorFlow pip package
2.TensorFlow with conda
Follow the similar steps as mentioned in above option or the use following one liner. This is quick and dirty way to start with your project with tensorflow -
conda create --name tensorflow-22 \
tensorflow-gpu=2.2 \
cudatoolkit=10.1 \
cudnn=7.6 \
python=3.8 \
pip=20.0
Alternatively, you can use the environment.yml file
name: null
channels:
- conda-forge
- defaults
dependencies:
- cudatoolkit=10.1
- cudnn=7.6
- nccl=2.4
- pip=20.0
- python=3.8
- tensorflow-gpu=2.2
Use environment.yml to create env insider your project.
conda env create --prefix ./env --file environment.yml --force
Q.5 How to use build TF from source?
Please follow the below article of mine to install tensorflow with following configuration -
Ubuntu 20.04
NVIDIA driver v450.57
CUDA 11.0.2 / cuDNN v8.0.2.39
GCC 9.3.0 (system default; Ubuntu 9.3.0-10ubuntu2)
TensorFlow v2.3.0
For a normally Python package, a simple pip install -U tensorflow but, unfortunately, TensorFlow is not that much simple in some configurations of hardware and dependencies. Sometimes after doing the pip install -U, errors of not founding cuda or other dependecies will be thrown.
Most of the I found that we won't get the precomplied versions of TF, with the configuration of machine I am using.
Most importantntly performance issues.
The official instructions on building TensorFlow from source are here: https://www.tensorflow.org/install/install_sources.
apt update
apt -y upgrade
sudo apt install build-essential libssl-dev libffi-dev python3-dev zip curl freeglut3-dev build-essential libx11-dev libxmu-dev libxi-dev libglu1-mesa libglu1-mesa-dev git software-properties-common librdmacm-dev
sudo apt install linux-image-extra-virtual linux-source linux-image-$(uname -r) linux-headers-$(uname -r)
wget -nc https://dl.winehq.org/wine-builds/winehq.key
sudo apt-key add winehq.key
sudo apt update
sudo apt-add-repository https://dl.winehq.org/wine-builds/ubuntu
sudo apt-add-repository main
lsmod | grep nouveau #if returns output then create below file to blacklist nouveau
sudo echo "blacklist nouveau" > /tmp/blacklist-nouveau.conf
sudo echo "options nouveau modeset=0" >> /tmp/blacklist-nouveau.conf
sudo cp /tmp/blacklist-nouveau.conf /etc/modprobe.d/
sudo update-initramfs -u #(rebuild the kernel)#install NVIDIA drivers
sudo add-apt-repository ppa:graphics-drivers/ppa
sudo dpkg -i ./nvidia-diag-driver-local-repo-ubuntu1604-384.183_1.0-1_amd64.deb
sudo apt-key add /var/nvidia-diag-driver-local-repo-384.183/7fa2af80.pub
sudo apt-get update
sudo apt-get install cuda_drivers
#install NVIDIA CUDA toolkit
sudo cuda_9.0.176_384.81_linux-run
sudo cuda_9.0.176.{X}_linux-run # X= 1,2,3,4 ...based on how many pacthes we have#install NVIDIA CUDNN7
sudo dpkg -i libcudnn7_7.6.5.32-1+cuda9.0_amd64.deb
sudo apt-get update
sudo apt-get install libcudnn7
sudo dpkg -i libcudnn7-dev_7.6.5.32-1+cuda9.0_amd64.deb
sudo apt-get update
sudo apt-get install libcudnn7-dev
#install python
curl https://bootstrap.pypa.io/get-pip.py -o get-pip.py && sudo python3 get-pip.py
sudo pip3 install virtualenv
virtualenv --system-site-packages -p python3 $RET/venv
source $RET/venv/bin/activate
#install NVIDIA tensorrt#download required tensorrt 6.0.1.5 and untar it
tar -xvzf TensorRT-6.0.1.5.Ubuntu-16.04.x86_64-gnu.cuda-9.0.cudnn7.6.tar.gz
cd TensorRT-6.0.1.5
sudo cp -R targets/x86_64-linux-gnu/lib /usr/lib/x86_64-linux-gnu/
sudo cp -R include/include/* /usr/include/
pip install uff/uff-0.6.5-py2.py3-none-any.whl
pip install graphsurgeon/graphsurgeon-0.4.1-py2.py3-none-any.whl
#setup TF
git clone https://github.com/tensorflow/tensorflow.git
git checkout r1.14.1
chmod +x bazel-0.24.1-installer-linux-x86_64.sh
./bazel-0.24.1-installer-linux-x86_64.sh --user
bazel build --config=opt --config=cuda //tensorflow/tools/pip_package:build_pip_package
./bazel-bin/tensorflow/tools/pip_package/build_pip_package ../tensorflow_pkg
pip install ../tensorflow_pkg/tensorflow-1.14.1-cp35-cp35m-linux_x86_64.whl
#setup Project specific env
sudo apt install python3-pil python3-pil.imagetk
pip install pillow
sudo apt-get install protobuf-compiler python-lxml
Set the environment variables.
export LD_LIBRARY_PATH=$LD_LIBRARY_PATH:/etc/alternatives
export LD_LIBRARY_PATH=$LD_LIBRARY_PATH:/usr/local/cuda/lib64
export LD_LIBRARY_PATH=$LD_LIBRARY_PATH:/usr/local/cuda/extras/CUPTI/lib64
export LD_LIBRARY_PATH=$LD_LIBRARY_PATH:/usr/lib/x86_64-linux-gnu/
Q.6 How to use GPUs from a docker container?
1. Official Docker images for the machine learning framework TensorFlow
You can download official TF images as per your need and use them.
2. Build from source with Docker 19.03
Versions earlier than Docker 19.03 used to require nvidia-docker2 and the --runtime=nvidia flag. Since Docker 19.03, you need to install nvidia-container-toolkit package and then use the --gpus all flag.
3. Third Party script
Use following article to build the tensorflow from sources and create docker container.
The author has provided, compilation images for Ubuntu 18.10, Ubuntu 16.04, CentOS 7.4, and CentOS 6.6.
Just need to check the bazel version for the from Tested build configurations.
LINUX_DISTRO="ubuntu-16.04"# or LINUX_DISTRO="ubuntu-18.10"# or LINUX_DISTRO="centos-7.4"# or LINUX_DISTRO="centos-6.6"cd "tensorflow/$LINUX_DISTRO"# Set env variablesexport PYTHON_VERSION=3.6
export TF_VERSION_GIT_TAG=v1.13.1
export BAZEL_VERSION=0.19
export USE_GPU=1
export CUDA_VERSION=10.0
export CUDNN_VERSION=7.5
export NCCL_VERSION=2.4
# Build the Docker image
docker-compose build
# Start the compilation
docker-compose run tf
# You can also do:# docker-compose run tf bash# bash build.sh
I hope you like this article. Please let me know what do you think about this in the comments. Suggestions/changes are welcome to make this article more inclusive and more informative.
Happy (Deep) Learning...! 😃
References -
Compile Tensorflow on Docker https://github.com/hadim/docker-tensorflow-builder
Building TensorFlow from source (TF 2.3.0, Ubuntu 20.04) https://gist.github.com/kmhofmann/e368a2ebba05f807fa1a90b3bf9a1e03
Installing the NVIDIA driver, CUDA and cuDNN on Linux (Ubuntu 20.04) https://gist.github.com/kmhofmann/cee7c0053da8cc09d62d74a6a4c1c5e4